КЛАСТЕРНИЙ АНАЛІЗ ДАНИХ ЖИТТЯ В ЄВРОПІ
DOI:
https://doi.org/10.31732/2663-2209-2020-58-40-51Ключові слова:
кластер, витрати на життя, місто, відстань, k-середні, k-медоїди, алгоритми ієрархічної кластеризаціїАнотація
Досить багато досліджень, резюме, доповідей та статей про вартість життя у різних містах світу. Незважаючи на те, що ці дослідження багаті та потужні, публікуються лише остаточні результати, такі як рейтинг міст або зведення найважливіших та найменш оцінених міст. Ця робота спрямована на вивчення найбільш придатних для життя міст у Європі за допомогою кластерного аналізу даних про ціни на життя за 2019 рік. Я застосую метод групування за подібністю або різницею в алгоритмах кластерного аналізу, оскільки існують багато методів кластерної кластеризації, і вони є технічними або алгоритмом (Пов'язування), де результати різні, використовуючи найпоширеніші методи зв'язування для складання дендрограми, а саме: мінімальне або індивідуальне зв'язування, максимальне або повне зв'язування, середнє або середнє зв'язування , де ми помітимо, що міста можуть згрупуватися в інший кластер відповідно до методу зв’язування та географічного розташування, де цей тип блокового аналізу робиться, щоб допомогти компаніям або навіть людям визначити, яке місто переїхати, щоб зменшити вартість життя. Також уникати прийняття неправильного рішення у разі зміни наступного кварталу для конкретного міста. Результати дозволяють описати подібність та відмінності в структурі цін на товари та послуги в різних містах та європейських країнах. Результати аналізу показали, що в деяких європейських країнах існують певні подібності у вартості життя та об’єднання їх в один кластер з урахуванням різниці в їх географічному розташуванні. Це вказує на те, що склад кластерів залежить від методу зв'язування в аналізі. Ці результати можуть бути використані приватними особами чи установами для вибору найкращих європейських країн для життя шляхом порівняння вартості життя в них порівняно з наявним бюджетом.
Завантаження
Посилання
Aboukadel, K. (2017), “Practical Guide To Cluster Analysis in R”. Unsupervised Machine Learning. Sthda Press. Edition 1.
Trevor, H. Robert, T. and Jerome, F. (2008), “The Elements of Statistical Learning”, Data Mining, Inference, and Prediction. Springer Press. Second Edition.
Sadanori, K. (2014), “Introduction to Multivariate Analysis”. Linear and Nonlinear Modeling Chapman & Hall Press.
. Richard, A. J. & Dean, W. W. (1998), “Applied Multivariate Statistical Analysis”, Prentice Hall Press. Four Edition.
Rafaela, C., D., M. & Alessandra, D., M. (2016), “The cost of living in the best livable cities in the world: a brief predictive quantitative analysis”. Int. J. Multivariate Data Analysis, Vol. 1, №1.
Numbeo (2019), “Is the world’s largest database of user contributed data about cities and countries worldwide”, Numbeo provides current and timely information on world living conditions including the cost of living, housing indicators, health care, traffic, crime, and pollution. 5,025,770 prices in 8,855 cities entered by 422,329 contributors and the information. Retrieved from: https://www.numbeo.com.
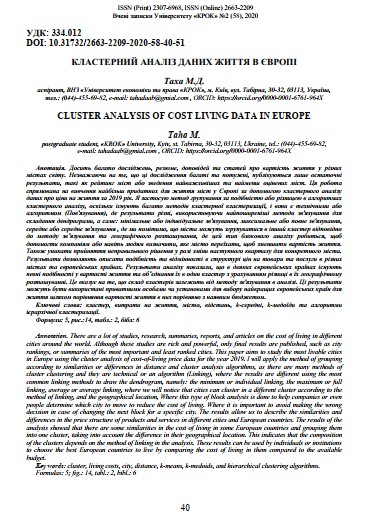